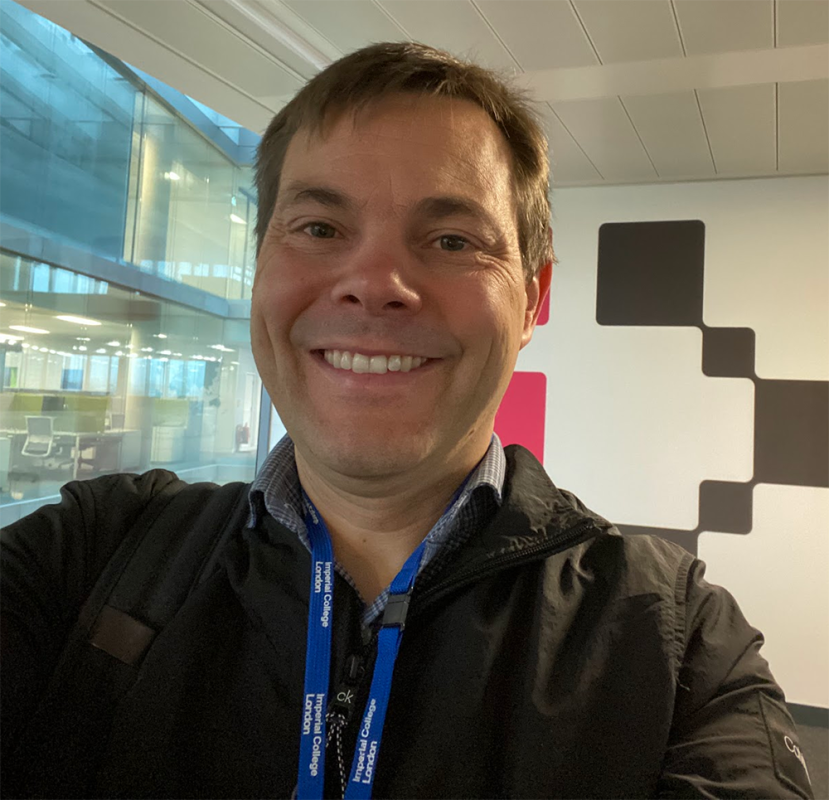
Department Seminar: Cédric John
Title: Planetary Transfer Learning: How Deep-Learning can be Leveraged to Advance our Understanding of Planets of the Solar System
Abstract: In this presentation I will go over the work done since 2022 in my research group, focusing on the use of satellite imagery and computer vision with deep-learning. I will mainly focus on applications on Earth, and how they can be leveraged to understand planetary processes on Mars. Over the last decades Mars has been the subject of multiple exploration campaigns led by several national space agencies. As a results, the planetary geoscience community now has a wealth of high-quality data from the surface of the red planet. This dataset can be used to help us understand wind patterns on Mars, an essential prerequisite to reconstruct Martian atmospheric patterns, predict surface storms and eolian sediment transport budget. However, only few direct in-situ measurements of wind strength and direction exist. Instead, satellite imagery of Mars can be used as a source of information on wind pattern. Here I show how we can combine the use of Earth analogues with the Mars Reconnaissance Orbiter high-resolution satellite images and deep-learning approaches.
In my group, we used images of eolian bedforms from Earth’ deserts to train two separate networks based on the Xception deep-learning architecture. Earth satellite images came from the Sentinel 5 satellite and were obtained using the Google Earth Engine API. Each image captures a 2.8x2.8 km square at a 10 m pixel resolution. Images were labelled using the daily wind strength and wind directions at 10 meters above Earth Surface averaged over a period of 10 years. We labelled images from sandy deserts and rocky terrain as ‘dune’ (image of wind-derived bedforms) and ‘no dune’ (no wind-derived bedform), respectively. Labelled were checked manually to ensure data quality. The dataset was split into a training set (80% of the images) and a validation and testing set (20% images) before data augmentation. Our final dataset contains over 480 thousand images after applying image augmentation (one symmetry and 3 rotations applied to the original dataset).
We first trained a classifier using the Xception architecture with a final layer containing one neuron activated with the sigmoid function. This resulted in a binary classifier that can recognize the presence of wind-derived bedforms with a precision of >94%. We then trained a regressor that uses the bedform geometry of images containing eolian bedforms to predict wind direction: we achieve better than a 10% error in the predicted direction of Earth winds based on sediment bedforms.
When applied to images from Mars, we obtain realistic looking patterns of winds. However, some images are clearly classified as dune, when they are not. In the second part of my work I will show more recent work from my team, where we explored style transfer learning as a mean to improve planetary transfer learning. We also explore AI explainability and a range of powerful algorithm that improve the overall accuracy of our classifier. Overall, our work shows how satellite images and Earth label images can offer a new way to understand other planets.